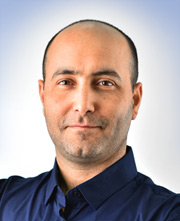
Benny Kimelfeld is a Professor in the Computer Science Faculty at Technion, Israel. He is the head and founder of the Technion Data and Knowledge (TD&K) Laboratory. He also belongs to the Faculty Network of RelationalAI. After receiving his Ph.D. from the Hebrew University of Jerusalem, and before joining Technion, he was a researcher at IBM Research Almaden, then a scientist at LogicBlox.
Benny’s research spans a spectrum of both foundational and systems aspects of data management, such as enumeration of query results, probabilistic, incomplete and inconsistent databases, infrastructure for text analytics, databases for preferences and social choice, and database aspects of machine learning. Benny was the program-committee chair of the 2018 International Conference on Database Theory (ICDT), a co-chair of the 2016 Web and Databases Workshop (WebDB), and a co-chair of the 2014 SIGMOD/PODS workshop on Big Uncertain Data (BUDA). He currently serves as an associate editor in the Journal of Computer and System Sciences (JCSS).
Research Interests: Uncertainty in Databases l Inconsistent Data Management | Text Analytics and Information Extraction l Enumeration Complexity l Preference Data Management | Databases & Machine Learning
I’ve had the great pleasure of giving the opening EDBT/ICDT 2020 keynote on “Facets of Probabilistic Databases.” The talk, as well as the entire conference, was given remotely via Zoom. See here more details about the content of the talk. (Yet, as fun as it was, I wish all of us a quick return to conferences in the real physical world.)
EDBT / ICDT 2018 Joint Conference, March 26-29, Vienna, Austria
Israel DB & IR Day 2017, June 19, Department of Computer Science, Technion